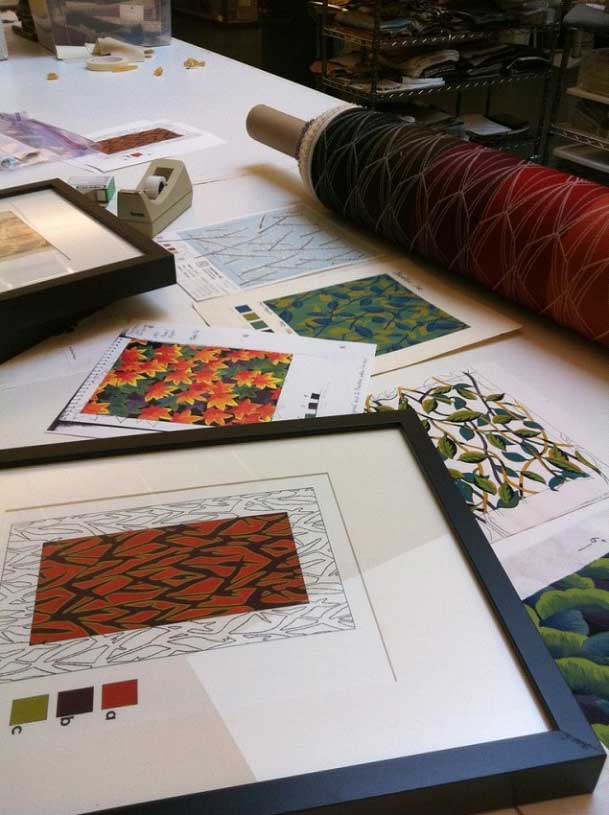
A comparative study of visual AI online systems from textile
The evolution of AI for visual creation has seen rapid advancements in recent years. Early AI visual creators were limited to simple tasks such as generating basic images or altering existing ones with predefined styles. However, recent developments in Generative Adversarial Networks (GANs) and Variational Autoencoders (VAEs) have created more complex and diverse visuals, including photorealistic images, animations, and even 3D models. These models are trained on large datasets and can generate new content based on patterns learned from the data. The technology continues to evolve, with new models and techniques being developed to improve the quality and versatility of AI-generated visuals.
Introduction to AI Visual Creator System (Online)
Artificial intelligence has revolutionised many fields, including computer vision and machine learning. The emergence of deep learning techniques has enabled the development of complex neural networks capable of generating realistic images. This literature review summarises the evolution of visual generative AI, starting from its earliest forms to the present state of the art.
The earliest form of visual-generative AI was introduced in the 1990s with the use of Markov models to generate images. These models used probability distributions to generate images, pixel by pixel. While they were able to generate simple images, they lacked the complexity and realism of modern generative models.
In the 2000s, researchers introduced the concept of deep belief networks (DBNs) for image generation. These networks were able to learn the probability distribution of images and generate new images by sampling from this distribution. While DBNs were an improvement over Markov models, they still lacked the ability to generate high-quality images.
One of the earliest examples of deep learning for visual generative AI is the autoencoder. An autoencoder is a neural network that learns to encode and decode images. The encoder network compresses the input image into a lower-dimensional representation, while the decoder network reconstructs the original image from this representation. Variational autoencoders (VAEs) introduced stochasticity into the encoding process, enabling them to generate new images by sampling from the learned distribution.
GANs are another type of deep learning network that has revolutionised visual generative AI. A GAN consists of a generator network and a discriminator network. The generator network learns to generate new images by sampling from a random noise distribution, while the discriminator network learns to distinguish between real and fake images. The two networks are trained in a competitive fashion, with the generator network trying to fool the discriminator network into thinking its images are real. GANs have been used to generate realistic images of faces, animals, and even entire landscapes.
Recent advances in visual-generative AI include the use of attention mechanisms to improve image generation. Attention mechanisms allow the generator network to focus on specific regions of the image, enabling it to generate images with more detail and complexity. Another recent advance is the use of style-based generators, which allow the user to control the style of the generated image by manipulating the input parameters.
Visual generative AI has come a long way since the early stages of Markov models. The introduction of deep learning techniques has enabled the development of complex neural networks capable of generating high-quality images. While there is still much to be done in terms of improving the realism and diversity of generated images, visual generative AI developments are progressing rapidly.
Methodology
In order to conduct a comparative study, the following methodology was used:
Out of the various available visual-based AI systems, three were selected to conduct the study. The key queries were: how can AI-based systems help textile designers create seamless patterns? Which AI supports the above objective, and what are the expected outputs and deliverables? What is the scope of visual generator AI systems to become a preferred tool for repeat pattern creation?
Based on popular styles of prints, key words were used to create repeat patterns of prints for each category in all three visuals.
In order to conduct a comparative study, the following methodology was used: AI systems. The possibilities and visual results were compared. A group of textile design students were asked to explore the AI tools and create visuals. A total of 60 visuals were generated and compared for analysis from a textile design perspective. Student feedback was taken as notes to determine the qualitative aspects. The research limited itself to exploring the free versions of all three Visual Creation AI online tools.
The evaluation was done based on quantitative as well as qualitative output generated by the AI. Therefore, the relative performance of the models was studied, documented, and presented through infographics. The paper discusses the strengths and limitations of each model and provides recommendations for textile design possibilities.
Textile design prompts
The field of visual-generative AI has rapidly progressed over the last decade. DALL-E 2, Stable Diffusion, and Adobe Firefly Beta are three of the latest and most exciting developments in this field. This comparative analysis explored these three models from a design perspective, comparing their strengths and limitations. In order to process the study, a basic data set was chosen. These data sets were instructional codes or textual prompts based on design and output requirements. The prompts were divided into two categories: fixed and variable. The variable prompts represented themes, creativity, colours, and style, while the fixed prompts were industrial parameters and design guidelines.
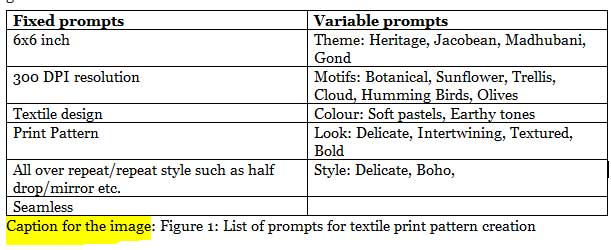
The first set of textile prints generation were based on the same set of key-words (Prompts) across all three Visual AI systems. Following is the first set of prompts that were used across Stable Diffusion, DALL E2 and Adobe Firefly Beta and the visual results were compared based on visual output, aesthetics, style and textile print pattern design parameters for textile designer’s perspective.
The first set of prompts: sunflower pastel colours humming bird and olives seamless repeat for textile print 6×6 inch 300 DPI resolution.
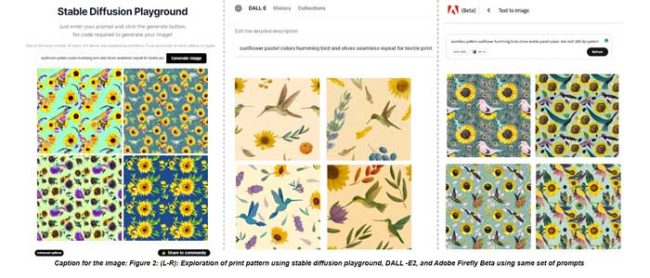
Comparative design perspective
DALL-E 2: DALL-E 2 is a generative AI model. It is capable of generating high-quality images from textual input, allowing users to specify the content, style, and composition of the image. One of the strengths of DALL-E 2 is its ability to generate highly detailed and complex images, such as those depicting imaginary animals or scenes. Additionally, DALL-E 2 can generate images that combine multiple concepts, such as an avocado-shaped chair.
However, DALL-E 2 has some limitations from a design perspective. One limitation is that it is primarily designed to generate single images rather than a series of images or animations. Additionally, while DALL-E 2 can generate highly complex images, it may not always produce the most aesthetically pleasing results.
Stable diffusion: Stable Diffusion is a generative AI model developed for image creation. It is designed to generate highly realistic and detailed images by iteratively refining a noisy initial image. One of the strengths of stable diffusion is its ability to generate highly detailed and photorealistic images, such as images of faces or landscapes.
Additionally, stable diffusion can generate images that combine multiple concepts or styles, allowing users to create highly unique and customised images. Another strength of stable diffusion is its ability to generate images in real-time, making it suitable for use in interactive applications or games.
However, one limitation of stable diffusion is that it may require significant computational resources to generate high-quality images, making it less suitable for use on low-end hardware. Additionally, stable diffusion may require more user input and intervention compared to other generative models.
DALL-E 2 and Stable Diffusion are three of the latest and most exciting developments in the field of visual generative AI. Each model has its own unique strengths and limitations from a design perspective, and choosing the right model will depend on the specific needs and goals of the user. Overall, these models represent a significant advancement in the fields of design and creative AI.
Fusing artistic intuition with cutting-edge algorithms empowers designers to craft intricate patterns effortlessly. Real-time adjustments ensure perfect alignment, while a user-friendly interface simplifies the process. From textiles to wallpapers, Stable Diffusion Beta reshapes pattern design, promising a future of boundless creativity.
Adobe Firefly Beta: Adobe Firefly Beta introduces a revolutionary toolset for effortless print pattern creation. It offers the blending of seamless patterns with an intuitive design ideation process. Firefly provides an open canvas for creative exploration for the designers with various features and value additions such as real-time adjustments, text effects, generative colors, text-to-image, and generative fill. For the comparative study, the “text-to-image tool” was explored.
DALL-E 2, Stable Diffusion, and Adobe Firefly Beta are three of the latest and most exciting developments in the field of visual generative AI. Each model has its own unique strengths and limitations from a design perspective, and choosing the right model will depend on the specific needs and goals of the user. Overall, these models represent a significant advancement in the fields of design and creative AI.
Analysis
Textile print creation is a challenging task that requires a high level of creativity and attention to detail. There are two aspects to any design creation: idea generation and real artwork creation for implementation.
In recent years, visual generative AI models such as Stable Diffusion Playground and DALL-E 2 have shown great potential for assisting designers in this process. In this comparative analysis, we will examine the strengths and weaknesses of Stable Diffusion, Adobe Firefly Beta, and DALL-E 2 for creating textile prints with seamless repeats based on textile print design: creative and technical specifications.
In order to create the images, the DALL-E 2 and Stable Diffusion Playground free versions were used. The enclosed chart is a comparative analysis from a textile print creation perspective.
DALL-E 2 is a transformer-based generative model that is capable of generating a wide range of images from textual input. It is designed to generate high-quality images that are semantically consistent with the input text. The model has been trained on a large dataset of images and can be fine-tuned to generate specific types of images, including textile prints.
In terms of strengths, Stable Diffusion Playground is highly flexible and can be fine-tuned to generate specific types of textile prints. The model is capable of generating a wide range of patterns and textures and can produce high-quality images that exhibit a high degree of diversity and realism. Additionally, Stable Diffusion Playground can generate images at high resolution, which is important for textile prints.
However, there are also some weaknesses associated with each model. Stable Diffusion may struggle to generate images that are semantically consistent with the input text, which can be a limitation for some designers.
DALL-E 2, on the other hand, may struggle to generate images that exhibit a high degree of diversity. This can be a limitation for textile print design, where a wide range of patterns and textures may be required. Additionally, the model may require a large amount of textual input to generate high-quality images, which can be time-consuming for designers.
Discussion
Stable Diffusion Playground, Adobe Firefly Beta, and DALL-E 2 have strengths and weaknesses when it comes to textile print creation. All three AI tools are useful for textile designers to experiment with and explore diverse visual aesthetics and inspiration. Despite motif creation and technically feasible production-friendly print pattern making, textile designers may still lean heavily towards industry-specific CAD/CAM systems. However, AI Visual Creator is a great tool to create mood boards, trend boards, story boards, and look boards. It is also useful for exploring visual aesthetics and experimenting with patchwork, mix-match, and hybrid styles of design inspiration. The free AI tools are amazing when it comes to finding inspiration and unusual combinations and visualisations of ideas instantly. The AI tools are very helpful in decoding various design and color trends and how industry and creative communities can use them effectively. AI gets better while creating more ideas as it learns during the process. It has the potential to act like a thinking partner and help with ideation during the design process.
References:
⦁ Bengio, Y. (2009). Learning deep architectures for AI. Foundations and trends® in Machine Learning, 2(1), 1-127.
⦁ Goodfellow, I., Pouget-Abadie, J., Mirza, M., Xu, B., Warde-Farley, D., Ozair, S., … & Bengio, Y. (2014). Generative adversarial nets. In Advances in neural information processing systems (pp. 2672-2680).
⦁ Hinton, G. E., & Salakhutdinov, R. R. (2006). Reducing the dimensionality of data with neural networks. Science, 313
⦁ OpenAI. (2021). DALL-E 2.⦁ https://openai.com/dall-e-2/
⦁ Adobe Research. (2021). Midjourney.⦁ https://research.adobe.com/project/midjourney/
⦁ OpenAI. (2021). Stable Diffusion.⦁ https://openai.com/blog/stable-diffusion/
⦁ Karras, T., Aila, T., Laine, S., & Lehtinen, J. (2018). Progressive growing of GANs for improved quality, stability, and variation. arXiv preprint arXiv:1710.10196.
⦁ Kynkaanniemi, T., & Kannala, J. (2021). Comparing deep generative models for realistic synthetic data generation. Pattern Recognition Letters, 151, 24-31.
⦁ https://labs.openai.com/
⦁ https://stablediffusionweb.com/
⦁ https://firefly.adobe.com/
About the author:
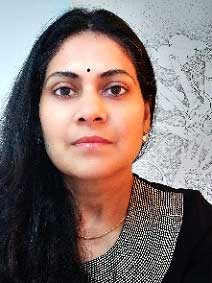
Dr. Kislaya Choudhary is a professor in the department of textile design and the present link. Chairperson for the Department of Textile Design, NIFT Mumbai She has a PhD in design and specialises in the textile design process. Her areas of exploration are conventional and digitally printed textiles, computer-aided design, interdisciplinary design research, contemporary craft practices, visual research, and design influence.